Can Machine Learning Translate Ancient Egyptian Texts?
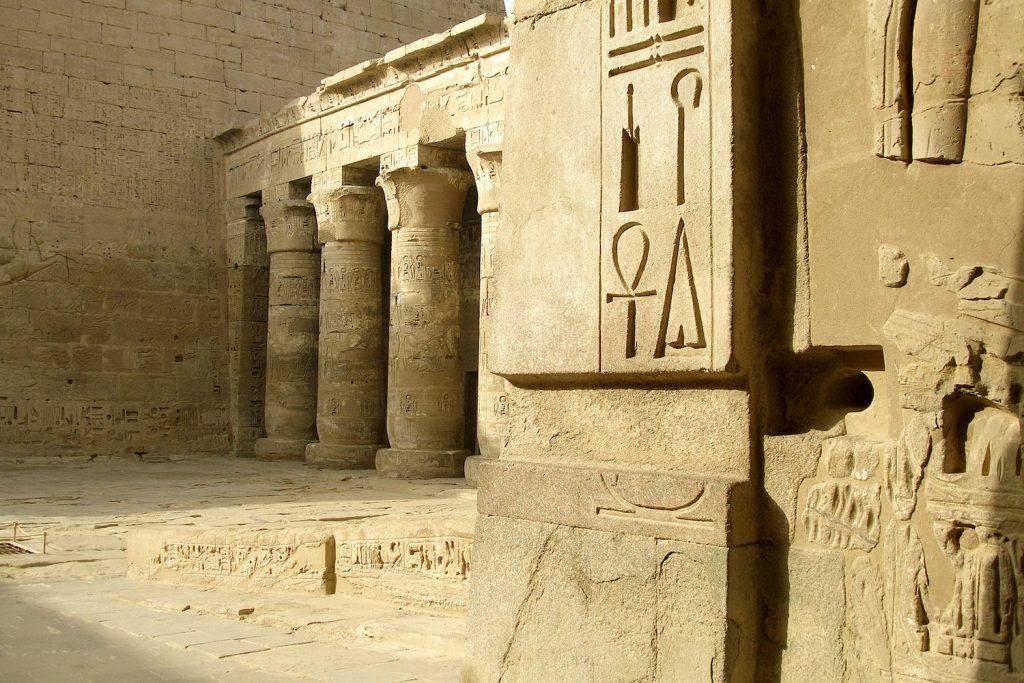
I have long been intrigued by archaeogaming—an academic discipline that explores the fusion of archaeological objects, methods, and characters into video games. So I was thrilled when the video game company Ubisoft released Assassin’s Creed: Origins, set in Egypt during Cleopatra’s reign. The designers collaborated with Egyptologists to ensure everything from the architecture to the hieroglyphics created an accurate, immersive world. Unexpectedly, this partnership inspired a machine-learning spinoff that changed the course of my early career.
While working with Egyptologists, the game developers learned that translating and interpreting ancient hieroglyphic texts is time-consuming, and the process has changed little in the last century. So, Ubisoft decided to give back to the academic community by developing the Hieroglyphics Initiative.
The project aims to help researchers decode Egyptian hieroglyphs using machine learning—a type of artificial intelligence that allows computer programs to become better at making predictions through experience.
The developers joined forces with a range of scholars within Egyptology, including members of the Australian Centre for Egyptology at Macquarie University, where I was a master’s student in search of a thesis topic. It felt like a sign. The Hieroglyphics Initiative was an opportunity for me to give back to the academic community by assisting with the development of a computer program that could benefit Egyptologists. I could explore how machine learning might transform archaeological research and education, and even how the public interacts with Egyptology.
The question was: Did the Hieroglyphics Initiative deliver on its promise? I made it my mission to find out.
The difficulties of deciphering hieroglyphs
Deciphering ancient Egyptian texts has challenged scholars for centuries. Ancient Egyptians regularly used more than 700 hieroglyphs, wrote their texts in different directions, and eschewed vowels, punctuation, and space between words. Many early researchers incorrectly assumed hieroglyphs were purely symbols; in fact, they can represent objects, ideas, or sound groups.
During the medieval era, Arab and Egyptian scholars preserved some knowledge of the hieroglyphic system, and European researchers were later informed by their efforts. But scholars in the West didn’t experience a major breakthrough until 1822, when Jean-François Champollion cracked the code by analyzing the multilingual inscription on the Rosetta Stone.
At first, translators used paper and pencil to sketch or trace inscriptions on tomb walls. Then in the 1890s, large-format photography revolutionized the field, allowing scholars to more accurately capture the details of inscriptions and reliefs.
The Hieroglyphics Initiative attempts to advance on these techniques by applying machine learning to capture and preserve this data. In 2020, Google Arts and Culture, which had taken over the project, released the program in English and Arabic, renaming it Fabricius.
Using this open-source program, researchers can upload a photo of text such as an inscription on a tomb wall, create a facsimile layer of the image, and zoom in on a specific section. They can also utilize the draw and erase function to touch up parts of the hieroglyphs that may be damaged by chinks in the rock wall.
Then they can sequence the hieroglyphs, and the program can help identify each hieroglyphic sign using Gardiner’s Sign List, which groups signs into categories such as birds, reptiles, or human body parts. Based on the sequence and identified hieroglyphs, the program can then suggest possible translations.
The process isn’t as simple or automatic as, say, the camera option on Google Translate, which translates text from images. It would be impossible for a machine, on its own, to fully incorporate all the nuances of ancient Egyptian grammar and spelling with enough accuracy to identify hieroglyphic sequences and suggest translations. This is because hieroglyphic signs can have multiple meanings, their spelling varies across thousands of years, and different scribes and artists had stylistic idiosyncrasies.
This means translation is complex and involves a high degree of interpretation. However, Fabricius could potentially help Egyptologists start a little further along the analytical process than they do when manually translating. And although Fabricius was initially designed with academics in mind, it has become available to the public, with the aim of making ancient Egyptian writing more accessible to the curious nonspecialist.
Read more about machine learning: “What If Machines Could Learn the Way Children Do?”
As part of my research, I created step-by-step instructions for using Fabricius and published articles that give nonexperts context about the history of decoding hieroglyphs and how Egyptologists are implementing various digital technologies into their research.
For example, Macquarie University Egyptologist Linda Evans examines animal imagery in ancient Egyptian art to better understand the area’s environmental history and the relationship between humans and other animals. She analyzed ancient Egyptian wall paintings using DStretch, a plugin that intensifies and saturates the colors in a digital photograph, allowing researchers to see faded details that are invisible to the eye. Using this technique, Evans was able to reinterpret several tomb paintings.
The work of Evans and others indicates that Egyptologists are interested in engaging with innovative new technologies and could potentially benefit from collaborations with companies such as Google and Ubisoft if projects like Fabricius prove successful.
can machines help translate hieroglyphs?
To investigate whether Fabricius is achieving its aims, I launched a case study, asking eight volunteers of varying levels of expertise to test the program. The participants came from Australia, Egypt, and the Netherlands, and ranged from students of Egyptology to career academics. Each participant was given two ancient Egyptian texts to translate—one using their usual method of translation, the other using Fabricius.
Initially, they were optimistic but also slightly skeptical about the program’s ambitious goals. Translation usually involves finding the sounds of particular signs in lists, seeking out words in dictionaries and online databases, using grammars to build good translations, and comparing translations of similar texts in various scholarly works, both analog and digital. This complex process is often carried out on paper and can take a number of attempts at scribbling, erasing, and crossing out symbols.
So, the idea that this multistep, laborious process could be simplified using machine-learning technology and completed on a single computer page intrigued the participants.
However, my study showed that Fabricius was not as immediately useful as initially hoped. The program lagged significantly, and its functions were not always self-evident to the users. This meant I had to guide each participant through the process to help them produce a translation in a timely manner. Most importantly, the machine-learning models used by Fabricius to suggest signs from traced photos achieved only a 27 percent accuracy rate.
That being said, the fact that the program can recognize and order hieroglyphic signs could make it a useful tool, even if recognition rates are not very high. Plus, machine learning allows programs to improve as they receive more and more data. Google Translate, which is powered by a machine-learning system, has become increasingly accurate over the years, and it performs best in languages it gets more training in, such as Spanish and German.
The glamour of machine learning might have to be put aside in favor of Fabricius’ pragmatic aspects.
So, as researchers create well-formed training data, a subsequent version of Fabricius could be trained on a much larger dataset. In this way, it could improve its accuracy in identifying hieroglyphs over time and help scholars prepare editions of Egyptian texts for use in a digital context, massively increasing the amount of textual data at their disposal for text and language research.
In short, Fabricius offers an opportunity to revolutionize the method of recording and conducting research into ancient Egyptian textual data. However, until the program evolves to a point where it could accurately identify at least 99 percent of signs, it is unlikely it could become a tool that supplements the manual procedures of textual analysis. Also, it could only be used as an accurate translation tool if the machine-learning module could be trained not just with individual signs (its current focus) but also with sentences and larger texts that incorporate grammar and syntax.
In the meantime, the glamour and mystique of machine learning might have to be put aside in favor of the more usefully pragmatic aspects of Fabricius’ design, such as the ability to quickly mark up and sequence all hieroglyphs within a text. This procedure alone would help digitize ancient Egyptian hieroglyphic texts at a much faster rate, allowing them to be easily shared, analyzed, and uploaded to online databases. Such databases could potentially create training information for future machine-learning applications.
In classrooms, Fabricius could generate a new learning mode for Egyptology students, encouraging emerging generations to develop skills that could improve the way hieroglyphic data is incorporated into an ever more technological world.
Overall, Fabricius represents a good first pass at building a program that can detect and automatically classify hieroglyphs. It needs retraining on a larger and better dataset before it could be used in a research context. However, the program holds promise as a platform for Egyptologists, students, and laypeople to engage with ancient texts and experiment with hieroglyphic translation. In addition, it might inspire more people to get involved with projects that combine archaeology, technology, and video games—as it did for me.